Call for papers
ENIAC 2024
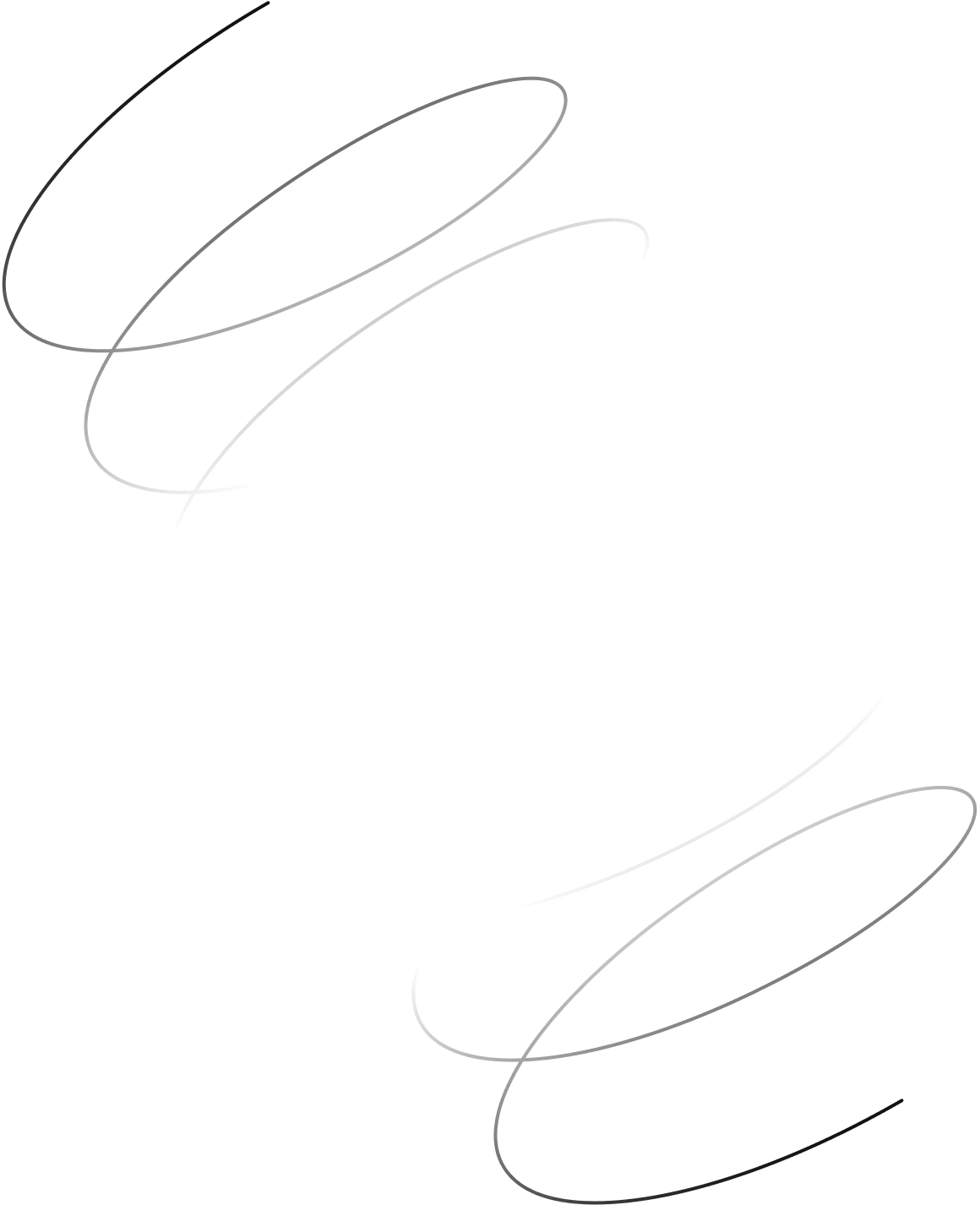
ENIAC 2024 is the 21st edition of a series of successful meetings bringing together Artificial Intelligence and Computational Intelligence, supported by Brazilian Special Interest Groups on Artificial Intelligence and Computational Intelligence from the Brazilian Computer Society.
ENIAC is part of the 34th Brazilian Conference on Intelligent Systems (BRACIS) to be held in Belém, PA, Brazil – from November 17th to 21st, 2024. This Brazilian meeting provides a forum for researchers, practitioners, educators, and students to present and discuss innovations, trends, experiences, and developments in the Artificial Intelligence and Computational Intelligence fields. In particular, it is the ideal event for postgraduate and undergraduate students to submit and present their ongoing results, even their first papers.
Plenary Speakers
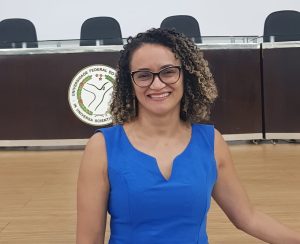
Eulanda Miranda dos Santos (IComp/UFAM)
———————————————————————————————————
Short Bio: EULANDA MIRANDA DOS SANTOS received a B.Sc. degree in Informatics from Federal University of Para (Brazil), a M.Sc. degree in Informatics from Federal University of Paraiba (Brazil) and a Ph.D. degree in Engineering at École de Technologie Supérieure, University of Quebec (Canada) in 1999, 2002 and 2008, respectively. Currently, she is a Full Professor at Federal University of Amazonas (Brazil). Her research interests include machine learning and computer vision. She develops R&D projects aimed at environmental protection and medical applications.
TITLE: INNOVATIONS AND CHALLENGES OF APPLICATION-DRIVENmachine learning: AN ANIMAL SPECIES CLASSIFICATION USE CASE
ABSTRACT: It is not unusual that machine learning methods considered state-of-the-art fail when used in real-world tasks. This happens because general methods are designed based on their performance on standardized benchmarks, while real-world data present several specific challenges. The focus of application-driven machine learning is developing methods to tackle a real-world problem, or a family of related problems, and their properties. Despite being focused on specific application tasks, application-driven machine learning may contribute to innovative methods, in turn advancing the state of the art in the entire research domain. In this talk, we will discuss the challenges and innovation possibilities of application-driven methods. We will present the problem of animal species classification in camera trap images as a use case to illustrate these issues more specifically.
Mauricio G. C. Resende, Ph.D. (UNIFESP-ITA PPG-PO and University of Washington) – S.J. dos Campos, Brazil and Seattle, USA
———————————————————————————————————
Short Bio: Mauricio G. C. Resende is and an Affiliate Professor of Industrial and Systems Engineering at the University of Washington and a Senior Professor of the Graduate Program in Operations Research of UNIFESP-ITA.
He grew up in Rio de Janeiro (BR), West Lafayette (IN-US), and Amherst (MA-US). He did his undergraduate training in electrical engineering (systems engineering concentration) at the Pontifical Catholic U. of Rio de Janeiro. He obtained an MS in operations research from Georgia Tech and a PhD in operations research from the U. of California, Berkeley.
He is most known for his work with metaheuristics, in particular GRASP and biased random-key genetic algorithms, as well as for his work with interior point methods for linear programming and network flows. Dr. Resende has published over 200 papers on optimization and holds 15 U.S. patents. He edited the Handbook of Heuristics (Springer, 2018), the Handbook of Optimization in Telecommunications (Springer, 2006), the Handbook of Massive Data Sets (Kluwer, 2002), and the Handbook of Applied Optimization (Oxford, 2002), and is coauthor of the book Optimization by GRASP (Springer, 2016). He has a Google Scholar h-index of 87 (over 33,500 Google Scholar citations) and is on the List of Top 2% Scientists of the World (2020-23). He sits on editorial boards of several optimization journals.
He worked at Amazon Research as a Principal Research Scientist in the logistics area from 2014 to 2022. Prior to Amazon, Resende was a Lead Inventive Scientist at the Mathematical Foundations of Computing Department of AT&T Bell Labs and at the Algorithms and Optimization Research Department of AT&T Labs Research from 1988 to 2014. Since 2016, he is Affiliate Professor of Industrial and Systems Engineering at the University of Washington in Seattle and since 1994, a Permanent Member of the Center for Discrete Mathematics and Theoretical Computer Science (DIMACS) at Rutgers University as well as a Senior Professor in the UNIFESP-ITA graduate program in operations research since 2023. He is a 2016 Fellow of INFORMS and was awarded the 2017 Constantine Carathéodory Prize.
TITLE: RANDOM-KEY OPTIMIZERS (RKO): PROBLEM INDEPENDENT COMBINATORIAL OPTIMIZATION
ABSTRACT: This talk introduces Random-Key Optimizers (RKO), a problem-independent approach for solving combinatorial optimization problems. Random keys are randomly generated real numbers in the interval (0,1]. A random-key vector is a vector of n random keys and corresponds to a point in n-dimensional unit hypercube. Solutions of combinatorial optimization problems can be encoded as random-key vectors. By using a decoder, a solution can be retrieved from a random-key vector. For example, by sorting a random-key vector, a permutation will result from the indices of the sorted vector. In a TSP these indices correspond to the cities in a tour. In the random-key optimization problem we seek a point in the n-dimensional unit hypercube that optimizes the decoder function. For a TSP, what is the point in the n-dimensional unit hypercube that decoded with the sorting decoder results in the shortest tour on the n cities. We illustrate this concept describing several decoders for different combinational optimization problems, and by presenting several RKOs, including a hybrid system that combines several RKOs into one intelligent system.
PAPER FORMAT AND SUBMISSION
Manuscripts are limited to twelve (12) pages including text, references, appendices, tables, and figures. Articles may be written either in Portuguese or English, using the SBC article style:
Papers written in Portuguese must have titles and abstracts in English. Manuscripts that do not follow the formatting guidelines might be rejected without review. Submissions should be carried out online using the JEMS system: https://jems.sbc.org.br/jems2/
Please select the appropriate track (Undergraduate track for papers whose main author is an Undergraduate student, Main track for the others).
The review process will be double-anonymous (authors’ names and institutions must be omitted in the papers). All submitted papers will be reviewed by at least two experts in the field. Authors of accepted papers will be invited to present their work either in an oral presentation or in a poster session. All accepted papers will likely be published electronically through the SBC Open Lib – SOL. There will be no distinction between papers presented in oral or poster format in the proceedings.
IMPORTANT DATES
- Submission deadline: August 5th, 2024
- Notification to authors: September 19st, 2024
- Camera-ready version: September 26th, 2024
REGISTRATION
TBA
SPECIAL ISSUES
Authors of the best papers will be invited to submit extended versions of their work to be appreciated for publication in special issues after the conference.
TOPICS OF INTEREST
The KDMiLe Program Committee invites submissions containing new ideas, proposals, and applications in the Data Mining and Machine Learning areas. Below is a list of common topics, although KDMiLe is not limited to them.
In Data Mining:
- Association Rules
- Classification
- Clustering
- Data Mining Applications
- Data Mining Foundations
- Evaluation Methodology in Data Mining
- Feature Selection and Dimensionality Reduction
- Graph Mining
- Massive Data Mining
- Multimedia Data Mining
- Multirelational Mining
- Outlier Detection
- Parallel and Distributed Data Mining
- Pre and Post Processing
- Ranking and Preference Mining
- Privacy and Security in Data Mining
- Quality and Interest Metrics
- Sequential Patterns
- Social Network Mining
- Stream Data Mining
- Text Mining
- Time-Series Analysis
- Visual Data Mining Web Mining
- Recommender Systems based on Data Mining
In Machine Learning:
- Active Learning
- Bayesian Inference
- Case-Based Reasoning
- Cognitive Models of Learning
- Constructive Induction and Theory Revision
- Cost-Sensitive Learning
- Deep Learning
- Ensemble Methods
- Evaluation Methodology in Machine Learning
- Fuzzy Learning Systems
- Inductive Logic
- Kernel Methods
- Knowledge-Intensive Learning
- Learning Theory
- Machine Learning Applications
- Meta-Learning
- Multi-Agent and Co-Operative Learning
- Natural Language Processing
- Probabilistic and Statistical Methods
- Ranking and Preference Learning
- Recommender Systems based on Machine Learning
- Reinforcement Learning
- Semi-Supervised Learning
- Supervised Learning
- Unsupervised Learning
- Online Learning
COMMITTEES
STEERING COMITTEE
Luiz Henrique de Campos Merschmann (UFLA)
Alexandre Plastino (UFF)
André Carlos Ponce de Leon Ferreira de Carvalho (ICMC-USP)
Wagner Meira Jr. (UFMG)
Ricardo Cerri (ICMC-USP)
PROGRAM CHAIR
- Rosiane de Freitas (IComp/UFAM) – rosiane@icomp.ufam.edu.br
- Diego Furtado (ICMC/USP) – diegofsilva@icmc.usp.br
PROGRAM COMMITTEE
TBA
IMPORTANT DATES (all deadlines are 11:59 p.m. UTC-12:00 – anywhere on Earth!)
– Deadline for submission: August 28, 2024
– Notification to authors: September 23, 2024
– Camera-ready versions due: October 05, 2024
ATTENTION
Generative AI models (including Chat-GPT, BARD, LLaMA, Gemini, etc.) or similar LLMs do not meet the article authorship criteria to be accepted into ENIAC 2024. However, we encourage articles that describe research on or involving such advanced AI models and tools.
If authors use an LLM in any part of the article writing process, they take full responsibility for all content, including checking for plagiarism and correcting all text.
TOPICS OF INTEREST
Authors are encouraged to submit articles containing new ideas, discussions on existing work, practical studies, and experiments relevant to the Artificial and Computational Intelligence fields, which have not been previously published. The topics of interest include, but are not limited to:
Analytics and Data Science
Artificial Life and Real-Time Simulation
Artificial Neural Networks and Deep Learning
Automated Planning, Routing and Scheduling
Automated Reasoning
Bioinformatics and AI in Medicine/Healthcare
Combinatorial Optimization
Complex Networks
Constraint Satisfaction Problem and Programming
Decision Support Systems
Distributed AI and Agent/Multiagent Systems
Education for AI and AI for Education
Embedded AI
Emerging Countries and Public Policies and AI
Evolutionary Computation and Metaheuristics
Explainable AI
Ethics and Human-centric AI
Foundation Models and Systems
Fuzzy Systems
Game Playing and Intelligent Interactive Entertainment
Generative AI
Graph-Based AI
Hybrid Systems and Metaheuristics
Intelligent Data Analysis
Intelligent Human-Computer Interfaces
Intelligent Systems
Intelligent Robotics
Knowledge Acquisition and Base Construction
Knowledge Representation and Reasoning
Large Language Models
Logic Programming
Machine Learning
Model-Based Reasoning
Multidisciplinary AI and CI
Natural Language Processing
Ontologies, Semantics, and Explanations
Pattern Recognition and Cluster Analysis
Quantum Computing and Learning
Reinforcement Learning
Software Tools for Artificial Intelligence
Temporal and Spatio-Temporal Data Mining
Time Series
Transformation of Work through AI
Transformers
Trustworthy Software and AI Systems
Vision AI
GENERAL CHAIR
André Ponce de Leon (ICMC/USP)
ENIAC 2024’s GENERAL/PROGRAM CHAIRS
Rosiane de Freitas (IComp/UFAM)
Diego Furtado (ICMC/USP)